
We are interested in the control of complex behaviours in medicine and the food industry using mechanistic (knowledge-based) mathematical models of microbiological relevant dynamics.
The challenge lays on increasing the predictive capabilities of such mathematical models combining theory with experiments.
Models and tools we have been working can be seen in GitHub
WE DEVELOP AND ADAPT DIFFERENT METHODOLOGIES LIKE
Microbiology lab
Including among others:
- Morbidostat: Automated continuous-culture device to determine the response of the bacterial population under dynamically sustained antimicrobial stress at different inocula .
- Flow cytometer
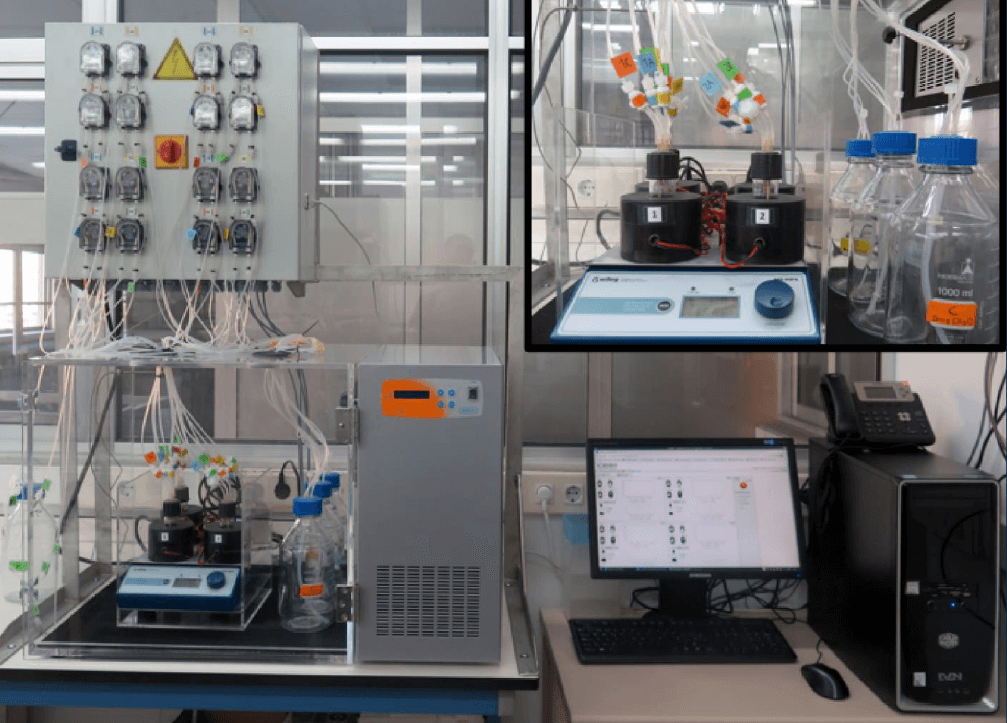
Model identification loop
Including for example:
- Parameter estimation and sensitivity analysis.
- Practical identificability analysis and confidence.
- Optimal experimental design.
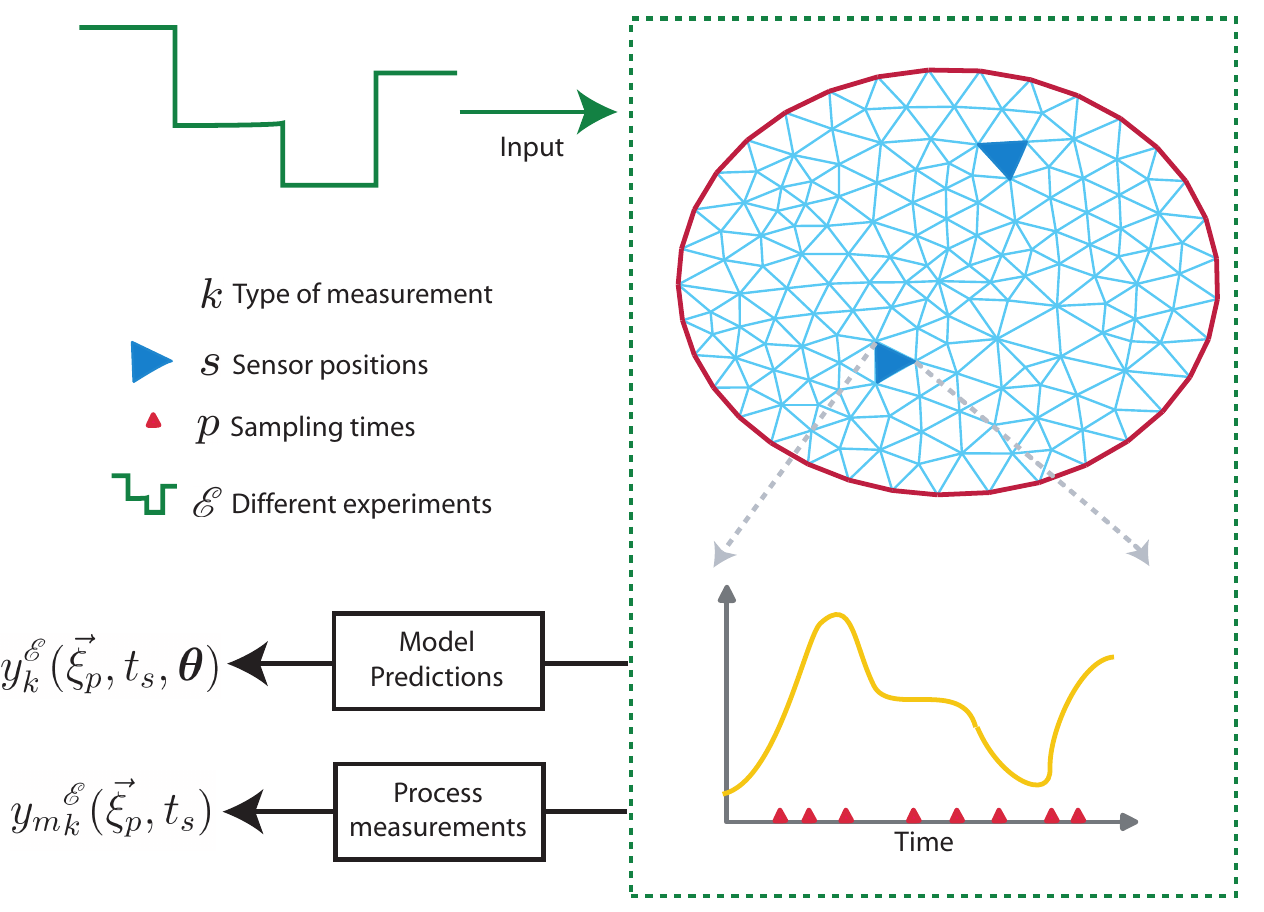
Parameter distributed systems
- Reaction-diffusion systems.
- Stochastic systems and the Fokker-Plank equation.
- Simulation and reduced order model, optimization and state identification.
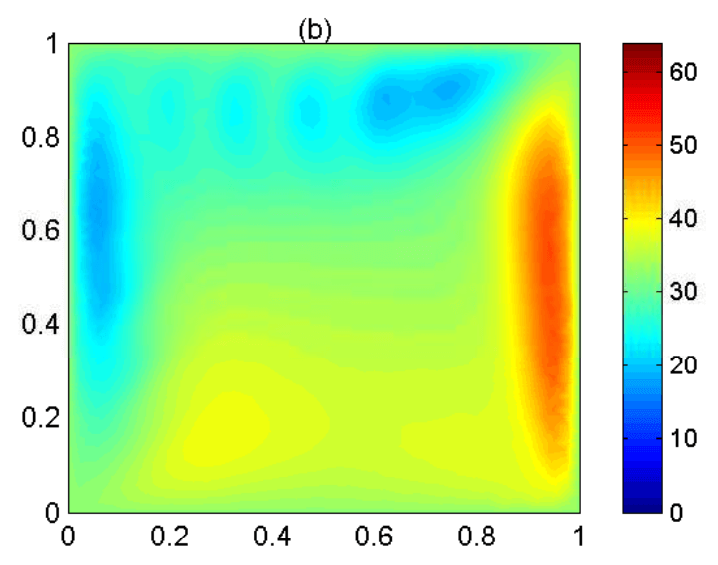
On-line optimization and control
- Real time optimization
- Model predictive control
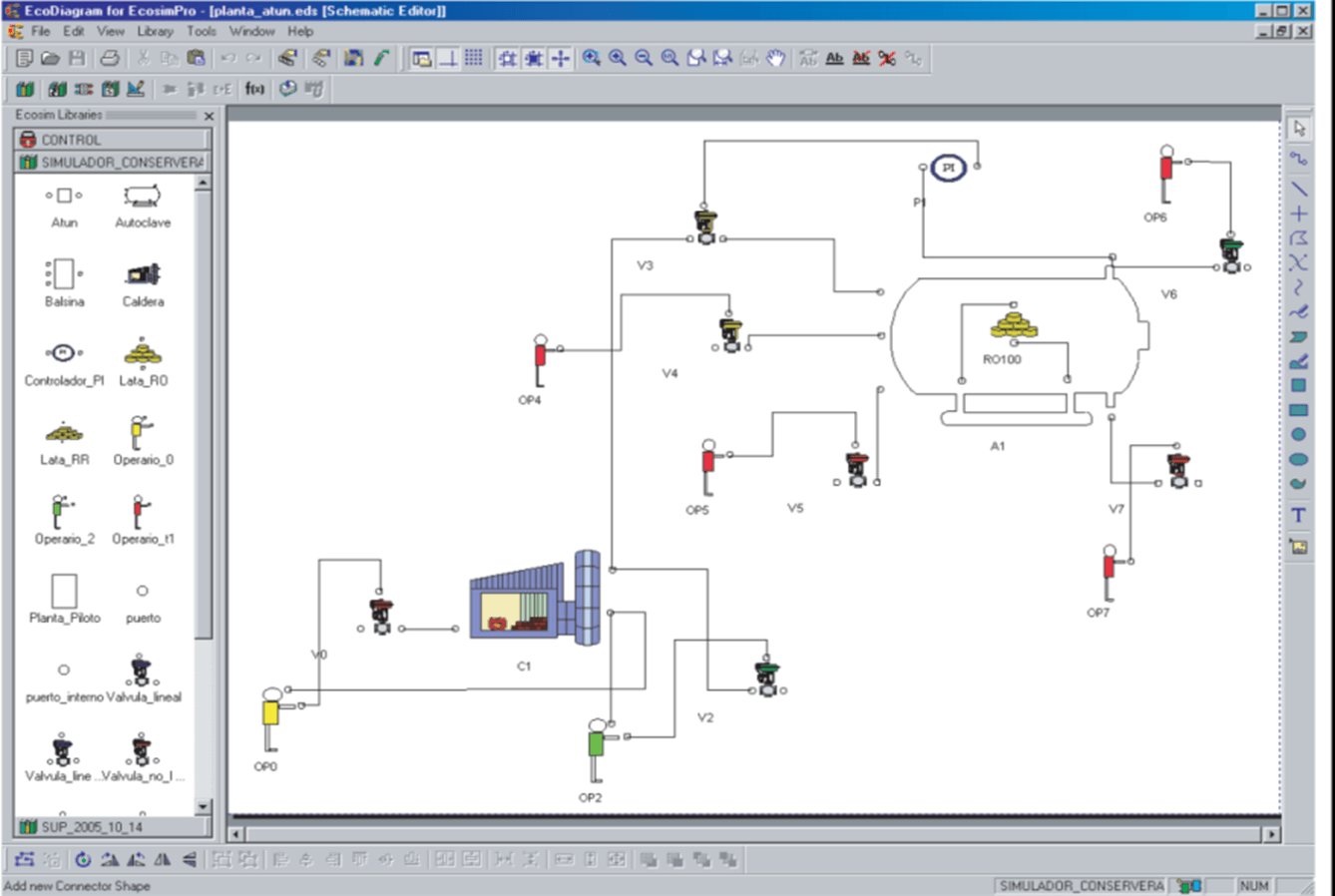
WE APPLY THE MODELS IN DIFFERENT AREAS
Food safety
For example the computation of the distribution and evolution of temperature for storage and transport of fishing products (EFSA Panel on Biological Hazards (BIOHAZ):
Koutsoumanis et. al. (2020) The use of the so‐called ‘tubs’ for transporting and storing fresh fishery products. Requestor: European Commission. Question number: EFSA-Q-2019-00053 EFSA Journal 18(4): . doi:10.2903/j.efsa.2020.6091.
OPEN CODE doi:10.5281/zenodo.3725616.
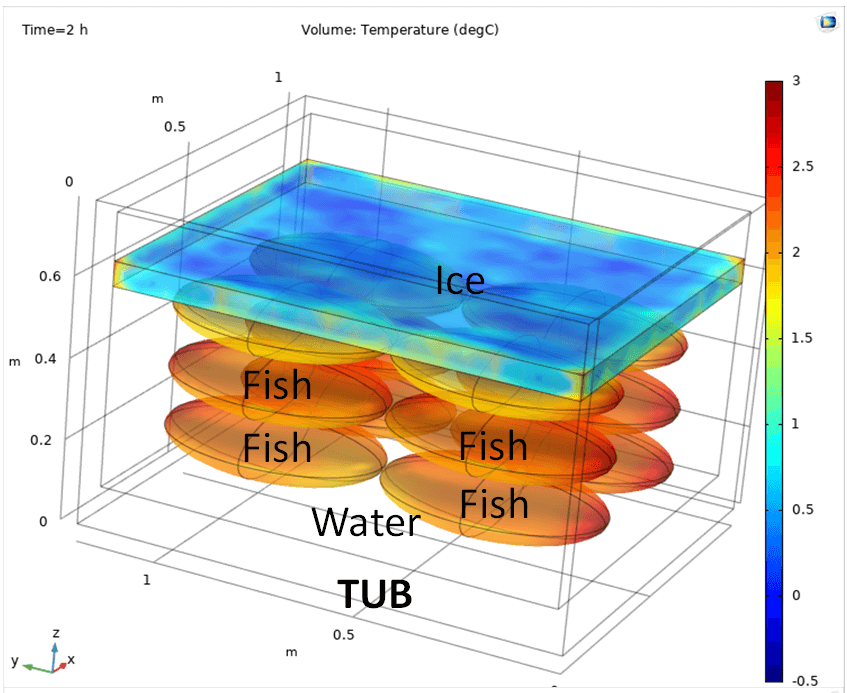
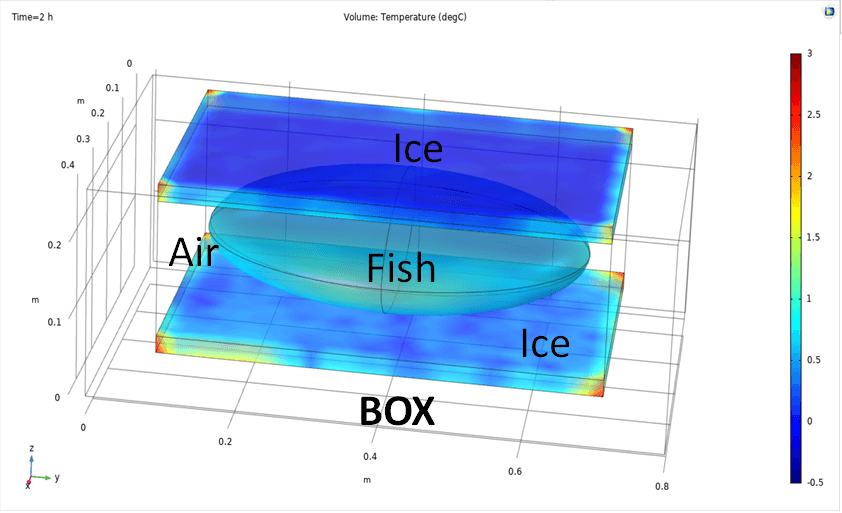
Food quality
For example the prediction of fish quality using mathematical models:
García, Férez-Rubio, Vilas (2022) Assessment and Prediction of Fish Freshness Using Mathematical Modelling: A Review. Foods 11(15): 2312. doi:10.3390/foods11152312.)
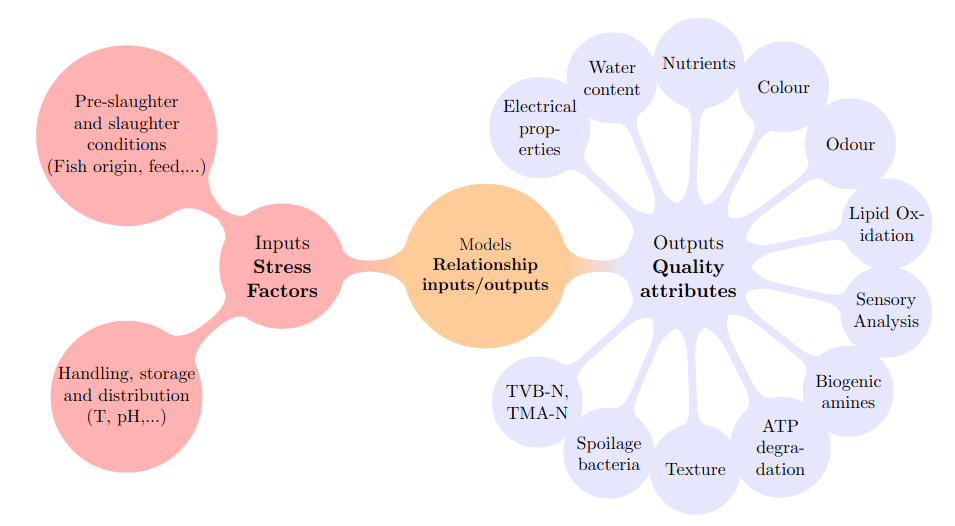
Medicine
For example the modelling of intracellular calcium dynamics
López-Caamal, Oyarzún, Middleton and García* (2014) Spatial quantification of cytosolic Ca2+ accumulation in nonexcitable cells: An analytical study IEEE/ACM Transactions on Computational Biology and Bioinformatics 11(3): 3592-603. doi:10.1109/TCBB.2014.2316010.
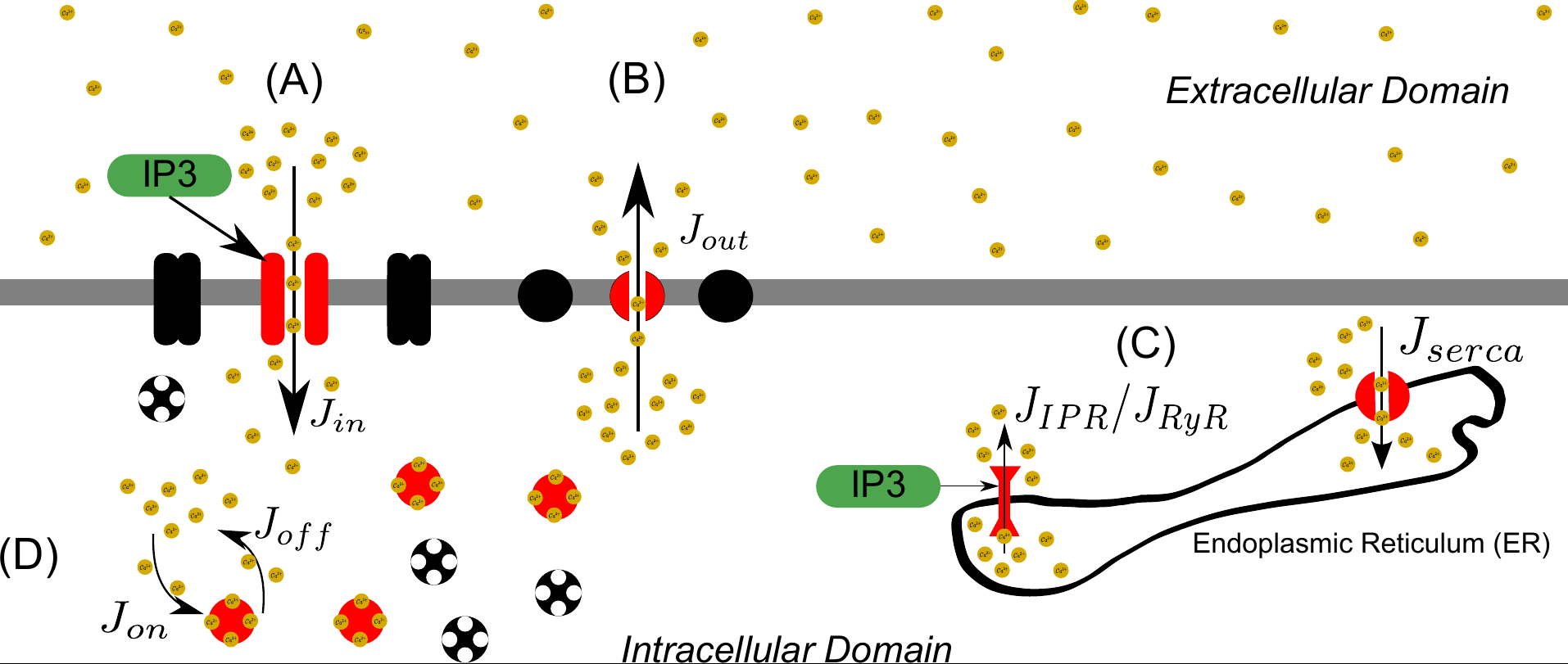